This blog post is contributed to Filecoin TL;DR by a guest writer. Catrina is an Investment Partner at Portal Ventures.
Until recently, startups led the way in technological innovation due to their speed, agility, entrepreneurial culture, and freedom from organizational inertia. However, this is no longer the case in the rapidly growing era of AI. So far, big tech incumbents like Microsoft-owned OpenAI, Nvidia, Google, and even Meta have dominated breakthrough AI products.
What happened? Why are the “Goliaths” winning over the “Davids” this time around? Startups can write great code, but they are often too hindered to compete with big tech incumbents due to several challenges:
- Compute costs remain prohibitively high
- AI has a reverse salient problem: a lack of necessary guardrails impedes innovation due to fear and uncertainty around societal ramifications
- AI is a black box
- The data “moat” of scaled players (big tech) creates a barrier to entry for emerging competitors
So, what does this have to do with blockchain technology, and where does it intersect with AI? While not a silver bullet, DePIN (Decentralized Physical Infrastructure Networks) in Web3 unlocks new possibilities for solving the aforementioned challenges. In this blog post, I will explain how AI can be enhanced with the technologies behind DePIN across four dimensions:
- Reduction of infrastructure costs
- Verification of creatorship and humanity
- Infusion of Democracy & Transparency in AI
- Installation of incentives for data contribution
In the context of this article,
- “web3” is defined as the next generation of the internet where blockchain technology is an integral part, along with other existing technologies
- “blockchain” refers to the decentralized and distributed ledger technology
- “crypto” refers to the use of tokens as a mechanism for incentivizing and decentralizing
Reduction of infra cost (compute and storage)
Every wave of technological innovation has been unleashed by something costly becoming cheap enough to waste
— Society’s Technical Debt and Software’s Gutenberg Momentby SK Ventures
The importance of infra affordability (in AI’s case, the hardware costs to compute, deliver, and store data) is highlighted by Carlota Perez’s Technological Revolution framework, which proposed that every technological breakthrough comes with two phases:
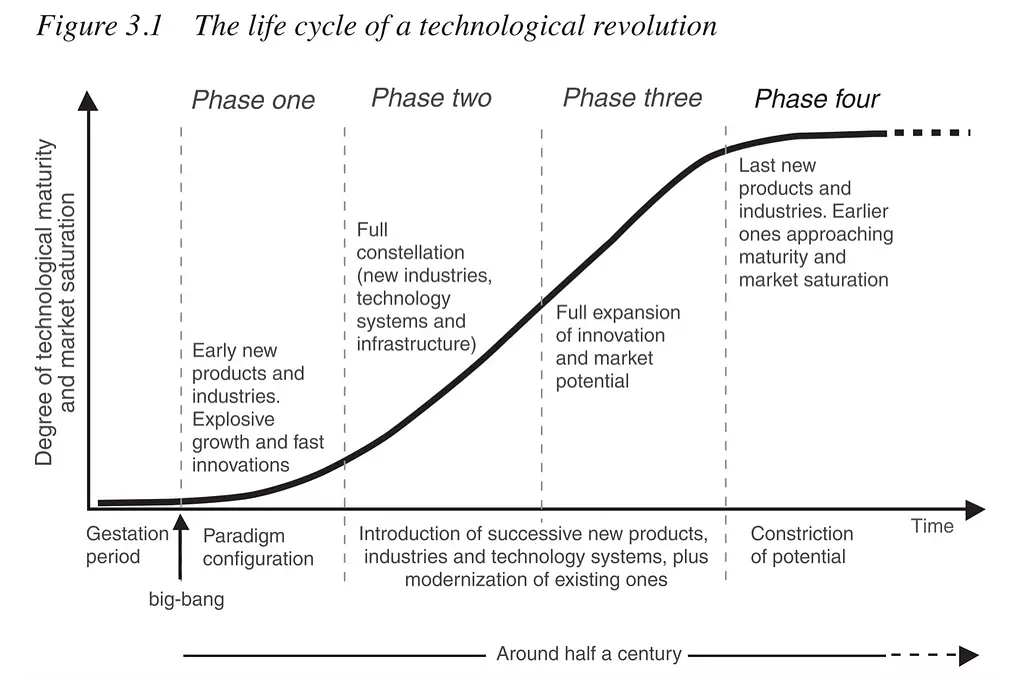
- The Installation stage is characterized by heavy VC investments, infrastructure setup, and a “push” go-to-market (GTM) approach, as customers are unclear on the value proposition of the new technology.
- The Deployment stage is characterized by a proliferation of infrastructure supply that lowers the barrier for new entrants and a “pull” GTM approach, implying a strong product-market fit from customers’ hunger for more yet-to-be-built products.
With definitive evidence of ChatGPT’s product-market fit and massive customer demand, one might think that AI has entered its deployment phase. However, there is still one piece missingstill: an excess supply of infrastructure that makes it cheap enough for price-sensitive startups to build on and experiment with.
Problem
The current market dynamic in the physical infrastructure space is largely a vertically integrated oligopoly, with companies such as AWS, GCP, Azure, Nvidia, Cloudflare, and Akamai enjoying high margins. For example, AWS has an estimated 61% gross margin on commoditized computing hardware.
Compute costs are prohibitively high for new entrants in AI, especially in LLM.
- ChatGPT costs an est. of 4M/training, and ~$700,000 per day to operate in hardware inference costs
- Version two of Bloom will likely cost $10M to train & retrain
- If ChatGPT were deployed into Google Search, it would result in $36B reduction in operating income for Google, a massive transfer of profitability from the software platform (Google) to the hardware provider (Nvidia)
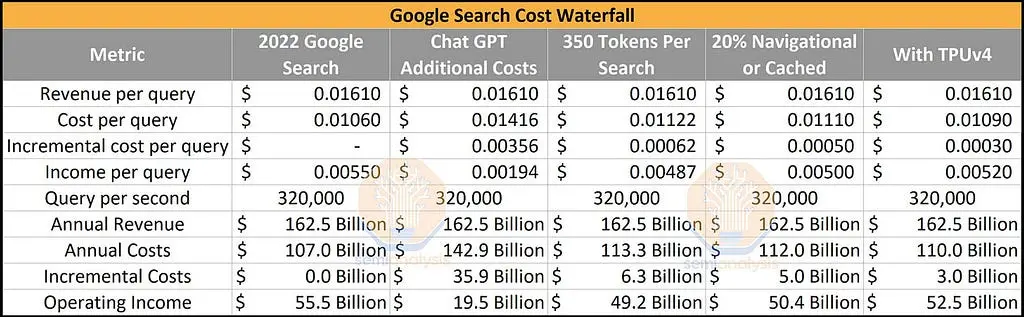
Solution
DePIN networks such as Filecoin (the pioneer of DePIN since 2014 focused on amassing internet-scale hardware for decentralized data storage), Bacalhau, Gensyn.ai, Render Network, and ExaBits (the coordination layers to match the demand for CPU/GPU with supply) can deliver 75% — 90%+ cost savings in infra costs via below three levers
1. Pushing up the supply curve to create a more competitive marketplace
DePIN democratizes access for hardware suppliers to become service providers. It introduces competition to these incumbents by creating a marketplace for anyone to join the network as a “miner,” contributing their CPU/GPU or storage power in exchange for financial rewards.
While companies like AWS undoubtedly enjoy a 17-year head start in UI, operational excellence, and vertical integration, DePIN unlocks a new customer segment that was previously out-priced by centralized providers. Similar to how eBay does not compete directly with Bloomingdale but rather introduces more affordable alternatives to meet similar demand, DePIN networks do not replace centralized providers but rather aim to serve a more price-sensitive segment of users.
2. Balancing the economy of these markets with crypto-economic design
DePIN creates a subsidizing mechanism to bootstrap hardware providers’ participation in the network, thus lowering the costs to end users. To understand how let’s first compare the costs & revenue of storage providers in web2 vs. web3 using AWS and Filecoin.
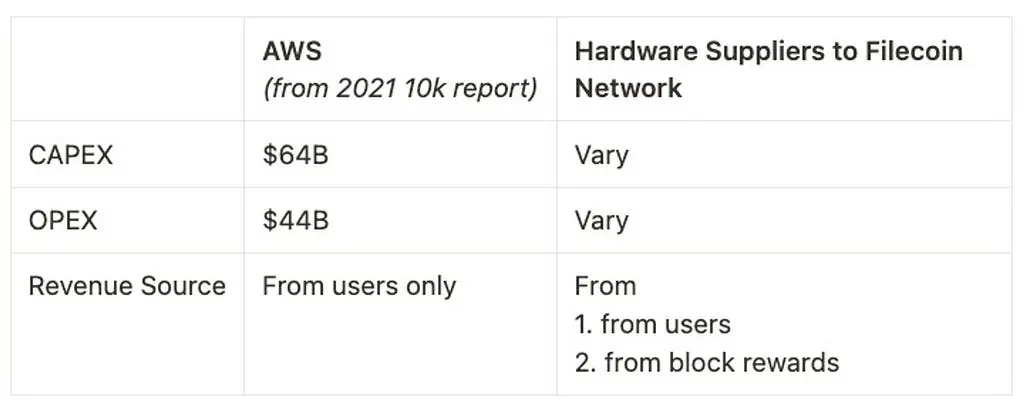
Lower fees for clients: DePIN networks create competitive marketplaces that introduce Bertrand-style competition resulting in lower fees for clients. In contrast, AWS EC2 needs a mid-50% margin and 31% overall margin to sustain operations, plus
Token incentives/block rewards are emitted from DePIN networks as a new revenue source. In the context of Filecoin, hosting more real data translates to earning more block rewards (tokens) for storage providers. Consequently, storage providers are motivated to attract more clients and win more deals to maximize revenue. The token structures of several emerging compute DePIN networks are still under wraps, but will likely follow a similar pattern. Examples of such networks include:
- Bacalhau: a coordination layer to bring computing to where data is stored without moving massive amounts of data
- exaBITS: a decentralized computing network for AI and computationally intensive applications
- Gensyn.ai: a compute protocol for deep learning models
3. Reducing overhead costs
Benefits of DePIN networks like Bacalhau and exaBITS, and IPFS/content-addressed storage include:
- Creating usability from latent data: there is a significant amount of untapped data due to the high bandwidth costs of transferring large datasets. For instance, sports stadiums generate vast amounts of event data that is currently unused. DePIN projects unlock the usability of such latent data by processing data on-site and only transmitting meaningful output.
- Reducing OPEX costs such as data input, transport, and import/export by ingesting data locally.
- Minimizing manual processes to share sensitive data: for example, if hospitals A and B need to combine respective sensitive patient data for analysis, they can use Bacalhau to coordinate GPU power to directly process sensitive data on-premise instead of going through the cumbersome administrative process to handle PII (Personal Identifiable Information) exchange with counterparties.
- Removing the need to recompute foundational datasets: IPFS/content-addressed storage has built-in properties that deduplicate, trace lineage, and verify data. Here’s a further read on the functional and cost efficiencies brought about by IPFS.
Summary by AI: AI needs DePIN for affordable infrastructure, which is currently dominated by vertically integrated oligopolies. DePIN networks like Filecoin, Bacalhau, Render Network, and ExaBits can deliver cost savings of 75%-90%+ by democratizing access to hardware suppliers and introducing competition, balancing the economy of markets with cryptoeconomic design, and reducing overhead costs.
Verification of Creatorship & Humanity
Problem
According to a recent poll, 50% of A.I. scientists agree that there is at least a 10% chance of A.I. leading to the destruction of the human race.
This is a sobering thought. A.I. has already caused societal chaos for which we currently lack regulatory or technological guardrails — the so-called “reverse salient.”
To get a taste of what this means, check out this Twitter clip featuring podcaster Joe Rogan debating the movie Ratatouille with conservative commentator Ben Shapiro in an AI-generated video.
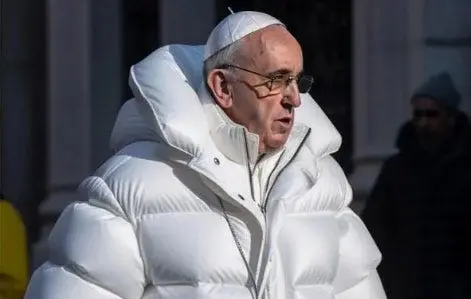
Unfortunately, the societal ramifications of AI go much deeper than just fake podcast debates & images:
- The 2024 presidential election cycle will be among the first where a deep fake AI-generated political campaign becomes indistinguishable from the real one
- An altered video of Senator Elizabeth Warren made it appear like Warren was saying Republicans should not be allowed to vote (debunked)
- The voice clone of Biden criticizing transgender women
- A group of artists filed a class-action lawsuit against Midjourney and Stability AI for unauthorized use of artists’ work to train AI imagery that infringed on those artists’ trademarks & threatened their livelihood
- A deepfake AI-generated soundtrack, “Heart on My Sleeve” featuring The Weeknd and Drake, went viral before being taken down by the streaming service. Such controversy around copyright violation is a harbinger of the complications that can arise when a new technology enters the mainstream consciousness before the necessary rules are in place. In other words, it is a Reverse Salient problem.
What if we can do better in web3 by putting some guardrails on AI?
Solution
Proof of Humanity and Creatorship with cryptographic proof of origination on-chain
This is where we can actually use Blockchain for its technology — as a distributed ledger of immutable records that contain tamper-proof history on-chain. This makes it possible to verify the authenticity of digital content by checking its cryptographic proof.
Proof of Creatorship & Humanity with Digital Signature
To prevent deep fakes, cryptographic proof can be generated using a digital signature that is unique to the original creator of the content. This signature can be created using a private key, which is only known to the creator, and can be verified using a public key that is available to everyone. By attaching this signature to the content, it becomes possible to prove that the content was created by the original creator — whether they are human or AI — and authorized/unauthorized changes to this content.
Proof of Authenticity with IPFS & Merkle Tree
IPFS is a decentralized protocol that uses content addressing and Merkle trees to reference large datasets. To prove changes to a file’s content, a Merkle proof is generated, which is a list of hashes that shows a specific data chunk in the Merkle tree. With every change, a new hash is generated and updated in the Merkle tree, providing proof of file modification.
A pushback against such a cryptographic solution may be incentive alignment: after all, catching a deep fake generator doesn’t generate as much financial gain as it reduces the negative societal externality. The responsibility will likely fall on major media distribution platforms like Twitter, Meta, Google, etc. to flag, which they are already doing. So why do we need Blockchain for this?
The answer is that these cryptographic signatures and proof of authenticity are much more effective, verifiable, and deterministic. Today, the process to detect deep fakes is largely through machine learning algorithms (such as the “Deepfake Detection Challenge”of Meta, “Asymmetric Numeral Systems” (ANS) of Google, and c2pa) to recognize patterns and anomalies in visual content, which is not only inaccurate at times but also falling behind the increasingly sophisticated deep fakes. Often, human reviewer intervention is required to assess authenticity, which is not only inefficient but also costly.
Imagine a world where each piece of content has its cryptographic signature so that everyone will be able to verifiably prove the origin of creation and flag manipulation or falsification — a brave new one.
Summary by AI: AI poses a significant threat to society, with deep fakes and unauthorized use of content being major concerns. Web3 technologies, such as Proof of Creatorship with Digital Signature and Proof of Authenticity with IPFS and Merkle Tree, can provide guardrails for AI by verifying the authenticity of digital content and preventing unauthorized changes.
Infusion of Democracy in AI
Problem
Today, AI is a black box comprised of proprietary data + proprietary algorithms. Such closed-door nature of Big Tech’s LLM precludes the possibility of what I call an “AI Democracy,” where every developer or even user should be able to contribute both algorithms and data to an LLM model, and in term receive a fraction of the future profits from the model (as discussed here).
AI Democracy = visibility(the ability to see the data & algorithm input into the model)
- contribution(the ability to contribute data or algorithm to the model).
Solution
AI Democracy aims to make generative AI models accessible for, relevant to, and owned by everyone. The below table is a comparison illustrating what is possible today vs. What will be possible, enabled by blockchain technology in Web3.
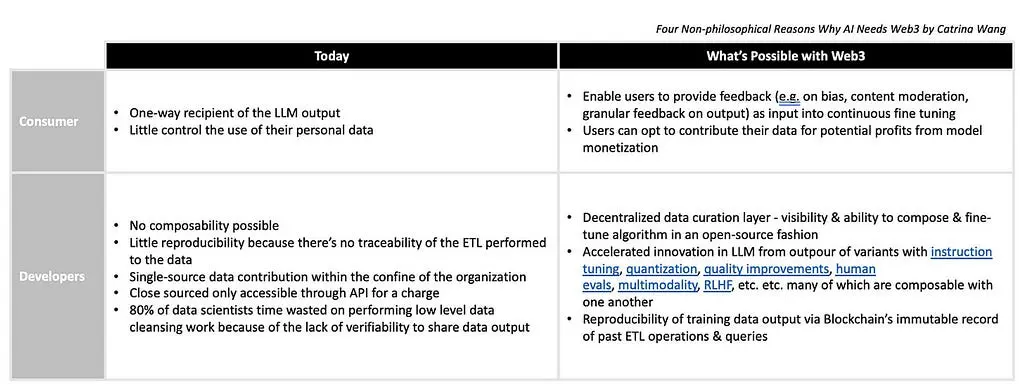
Today:
For consumers:
- One-way recipient of the LLM output
- Little control over the use of their personal data
For developers:
- Little composability possible
- Little reproducibility because there’s no traceability of the ETL performed on the data
- Single-source data contribution within the confine of the owner organization
- Close sourced only accessible through API for a charge
- 80% of data scientists’ time is wasted on performing low-level data cleansing work because of the lack of verifiability to share data output
What blockchain will enable
For consumers:
- Users can provide feedback (e.g. on bias, content moderation, granular feedback on output) as input into continuous fine-tuning
- Users can opt to contribute their data for potential profits from model monetization
For developers:
- Decentralized data curation layer: crowdsource tedious & time-consuming data preparation processes such as data labeling
- Visibility & ability to compose & fine-tune algorithms with verifiable & built-on lineage (meaning they can see a tamper-proof history of all changes in the past)
- The sovereignty of both data (enabled by content-addressing/IPFS) and algorithm(e.g. Urbitenables peer-to-peer composability and portability of data & algorithm)
- Accelerated innovation in LLM from the outpour of variants from the base open-source models
- Reproducibility of training data output via Blockchain’s immutable record of past ETL operations & queries (e.g. Kamu)
One might argue that there’s a middle ground of Web2 open source platforms, but it’s still far from optimal for reasons discussed in this blog post by exaBITS.
Summary by AI: The closed-door nature of Big Tech’s LLM precludes the possibility of an “AI Democracy,” where every developer or user should be able to contribute both algorithms and data to an LLM model, and in turn receive a fraction of the future profits from the model. AI should be accessible for, relevant to, and owned by everyone. Blockchain networks will enable users to provide feedback, contribute data for potential profits from model monetization, and enable developers to have visibility and the ability to compose and fine-tune algorithms with verifiability and built-on lineage. The sovereignty of both data and algorithm will be enabled by web3 innovations such as content-addressing/IPFS and Urbit. Reproducibility of training data output via Blockchain’s immutable record of past ETL operations and queries will also be possible.
Installation of Incentives for Data Contribution
Problem
Today, the most valuable consumer data is proprietary to big tech platforms as an integral business moat. The tech giants have little incentive to ever share that data with outside parties.
What about getting such data directly from data originators/users? Why can’t we make data a public good by contributing our data and open-source it for talented data scientists to use?
Simply put, there’s no incentive or coordination mechanism for that. The tasks of maintaining data and performing ETL (extract, transform & load) incur significant overhead costs. In fact, data storage alone will become a $777 billion industry by 2030, not even counting computing costs. Why would someone take on the data plumbing work and costs for nothing in return?
Case in point, OpenAI started off as open-source and non-profit but struggled with monetization to cover its costs. Eventually, in 2019, it had to take the capital injection from Microsoft and close off its algorithm from the public. In 2024, OpenAI is expected to generate $1 billion in revenue.
Solution
Web3 introduces a new mechanism called dataDAO that facilitates the redistribution of revenue from the AI model owners to data contributors, creating an incentive layer for crowd-sourced data contribution. Due to length constraints, I won’t elaborate further, but below are two related pieces.
- How DataDAO works by HQ Han of Protocol Labs
- How data contribution and monetization works in web3, where I dove into the mechanics, missing pieces, and emerging inevitable opportunities in dataDAOs
In conclusion, DePIN is an exciting new category that offers an alternative fuel in hardware to power today’s renaissance of innovations in web3 and AI. Although big tech companies have dominated the AI industry, there is potential for emerging players to compete by leveraging blockchain technologies: DePIN networks lower the barrier to entry in compute costs; blockchain’s verifiable & decentralized properties make true open IA possible; innovative mechanisms, such as dataDAOs, incentivize data contribution; and the immutable and tamper-proof property of Blockchain provides proof of creatorship to address concerns regarding the negative societal impact of AI.
Some good resources for DePIN deep dive
Shoutout to below SMEs and friends for providing input and/or reviewing my draft: Jonathan Victor& HQ Han of Protocol Labs, Zackary Bof exaBITS, Professor Daniel Rock of Wharton, Evan Fisherof Portal Ventures, David Aronchickof Bacalhau, Josh Lehmanof Urbit, and more
About Author: Partner at Portal Ventures | @CuriousCatWang on Twitter